Wind energy offers enormous potential for renewable energy generation as
part of the clean energy transition necessary to reduce the production of damaging carbon emissions, while fulfilling demand for increasing
quantities of affordable and reliable energy. A wind farm is a collection of wind turbines. For our purposes, consider a 3×3 grid of 9 wind turbines. The freestream wind speed and direction incident upon the wind farm describe the wind field outside of the collection of wind turbines, before it is, for want of a better word, mangled within the farm. The reason for this ‘wind mangling’ is that once the freestream wind passes through the upstream turbines (those that partially shield other turbines in the wind farm from wind), wakes propagate downstream, causing lower wind speeds and higher turbulence at downstream turbines, reducing power production and increasing mechanical loads (see the figure below).
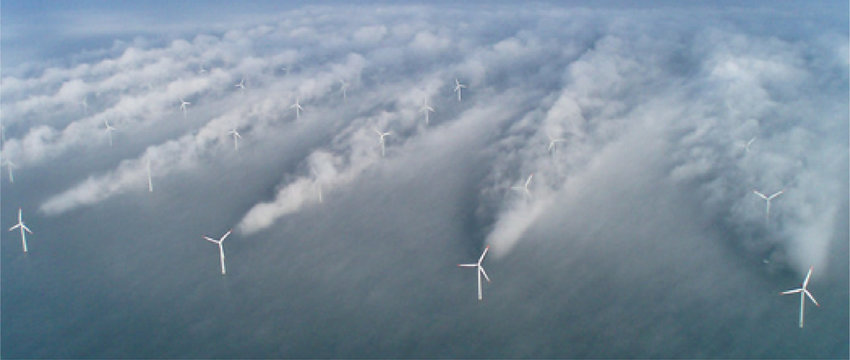
Typically, the purpose of wind farm control is to change the yaw angles of the individual wind turbines (their rotation about a vertical axis):
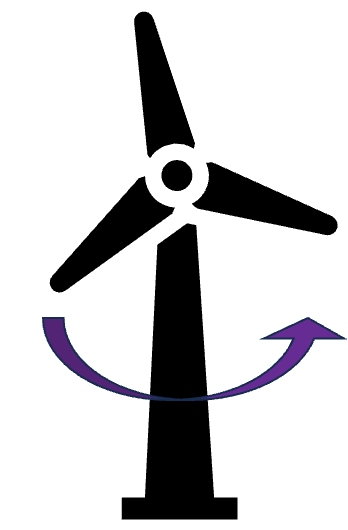
… so as to optimally redirect the wake of upstream turbines, with the objective of maximizing the total power output of this collection of wind turbines, while minimizing their peak mechanical loads, in response to time-varying freestream wind speed and direction. Receding horizon model-based control methods (i.e. strategies to automatically adjust the yaw angles to reach the above objectives), require a sufficiently accurate means of predicting the influence of the wakes within a wind farm over some future time-horizon. In particular, if we have a means of making sufficiently accurate predictions of the wind speed at downstream wind turbines over the next 1 to 10 minutes, we can optimally choose the best yaw angle set-points for each turbine to diminish the effect of the wakes and improve farm-level power production and turbine damage.
Existing options for models describing the wind speed at downstream wind turbines in wind farms include low-fidelity, analytical models, or high-fidelity models that can resolve dynamics and turbulence, such as large-eddy simulations (LES). The problem with the former is that these simplified analytical models a) are unlikely to capture all of the important variations in the wind farm wind speeds we are trying to predict and b) generally do not have the capacity to account for freestream wind speed and direction variability, or variability in the wind farm layout (i.e. due to one or more wind turbines being turned off for maintenance purposes, due to a malfunction, or because they are producing more energy than is needed at the time). The problem with the latter is that such high-fidelity models are too computationally expensive to use for real-time controls purposes.
The current ‘state-of-the-art’ wind farm control strategy involves utilizing low-fidelity steady-state models and optimizing the yaw angles of every turbine in the wind farm for a sweep of freestream wind speeds and directions. There are two issues with this. Firstly, real wind farm operation is anything but steady-state: the mean values of freestream wind speed and direction vary with time and also vary over the area covered by the wind farm, and in addition turbulence contributes to greater time variability. Secondly, this ‘offline optimization’ method assumes full capacity wind farm operation, in which every turbine is ‘online’ (i.e. fully operational and harvesting energy from the wind). The reality is that, for a significant proportion of a wind farm’s life, at least one turbine is ‘offline’ for anything from hours to months. This could be because power from the farm is being curtailed, since more is being produced than is in demand by the power grid at that time, because the turbine is malfunctioning, or because the turbine is in need of maintenance. In any case, the wind field in partially operational wind farms will certainly differ from that in a fully operational wind farm, but the offline optimization approach only assumes the latter.
The final objective of this project, while not achievable within the given timeline, is to develop a machine learning strategy to predict the wind speeds at downstream wind turbines in a wind farm over a 10 minutes future time horizon, given measurements of yaw angles and wind speeds from the other turbines over the previous 10 minutes. as well as the freestream wind speeds and directions over that time. The most useful strategy could be updated in real-time (online) to account for time-varying wind speeds, wind directions and wind farm layouts. This machine learning model could then be integrated into a control method to choose the optimal yaw angles for the turbines in real-time such that a) wind farm power generation is maximized, b) wind turbine damage is minimized, and ultimately so that c) wind energy becomes a drastically more viable alternative energy source to oil/coal/gas.